people
A data scientist for the aviation industry of tomorrow
He analyzes, decodes and finds connections. Data scientist Dr. Oliver Arnold manages the interface between information technology and manufacturing technology.
06.2019 | author: Thorsten Rienth | 3 mins reading time
author:
Thorsten Rienth
writes as a freelance journalist for AEROREPORT. In addition to the aerospace industry, his technical writing focuses on rail traffic and the transportation industry.
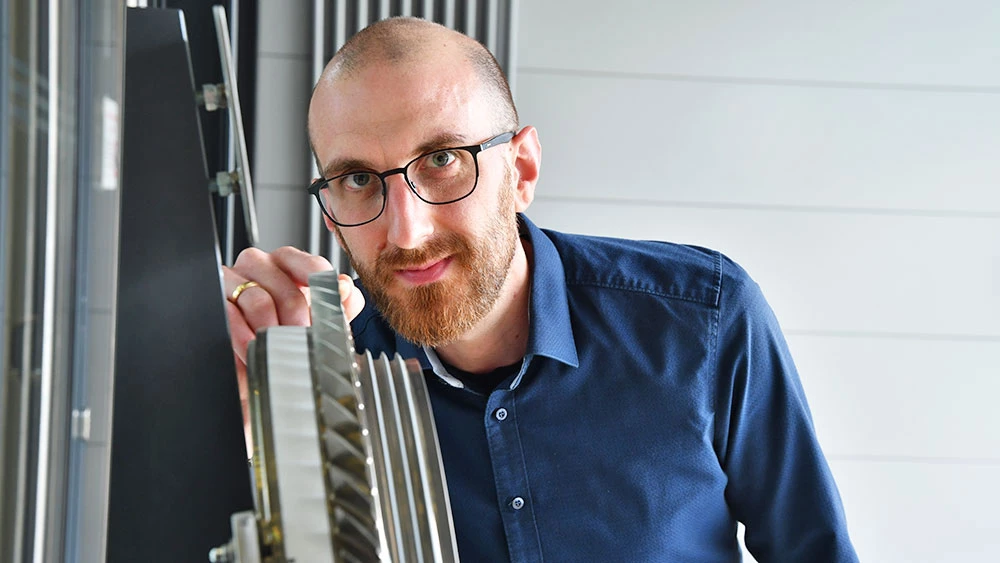
In the engine manufacturing business, there is nothing more important than high-quality components. Take blisks, for example: in these high-tech components that integrate blade and disk, even a hundredth of a millimeter can make all the difference. In the worst case, the entire component may end up being worth only as much as the materials used to make it. Following current procedures, production engineers cannot be sure a blisk meets specifications until after the final inspection, long after the hours-long machining process has been completed.
- Using multiphysics simulations to boost efficiency
- A data scientist for the aviation industry of tomorrow
- Digitalization paves the way for predicting tool wear
- Managing digital transformation
- Digital tools help create new, efficient components
- Talking geometries: A common language for more efficient engine development
What if it were possible to detect critical deviations much earlier on? For example, what if it were possible to react to signs of a tolerance deviation during the manufacturing process? Then it would be possible to avoid extensive and costly reworking.
Data that is produced, accessed or processed by manufacturing machinery during the production process can play a decisive role in finding a solution. But there is a huge quantity of information. To be able to utilize it, someone has to determine which information is relevant. This person analyzes the data, decodes it, and finds connections.
For blisk manufacturing at MTU Aero Engines, Dr. Oliver Arnold is that person. A 34-year-old physicist and data researcher, Arnold left a position at CERN, the European Organization for Nuclear Research, about a year ago for his current job in Munich. In the canton of Geneva he had worked on high-energy experiments in basic physics research. At MTU he wants to create algorithms that bundle valuable data related to the extremely complex blisk manufacturing process.
“In plain words, we take a look at the machine data that is produced in the process of machining the blisks,” Arnold explains. “We try to detect patterns and interdependencies in the data that may be reflected in substandard component quality, which doesn’t become apparent until later in the manufacturing process.” The extraction of reliable trends would provide production engineers with a solid foundation for their decision-making, enabling them to react at an earlier stage, improve processes and cut costs.
Achieving this goal is not as easy as it sounds. How can the truly relevant information be extracted from the enormous quantities of data supplied by the machines? How often would it be advisable to capture the data? What level of data quality is needed to determine which causalities? And what additional sensors might be urgently needed in the future—and should thus already be included in the specifications for new machinery that is about to be procured? “We are still at a relatively early stage,” says Arnold. “But we are making our way toward the solutions step by step.”
The development of a completely data-driven understanding of processes is still a very new science. For example, the Technical University of Munich (TUM) was one of the very first universities to offer a master’s degree in data science, introducing it in 2015. Four years on, professionals in this field are now indispensable in every high-tech industry.
“The fascinating thing about this for me is definitely the interdisciplinary approach,” says Arnold. “I myself cannot comprehend the data without help from my colleagues involved in the manufacturing process. They provide the process expertise and together we transform it—along with the data—into a model.” One could view Arnold as an interpreter: at the interface between information technology and manufacturing strategy, his analysis provides support for the decision-making process.